Curator Informed Models
AI-Driven Curation of Measurement Data
Concept
At Compolytics, we are pioneering the development of curator-informed models, an innovative approach to curating measurement data using artificial intelligence (AI). This research is driven by the increasing complexity of modern sensor data, where quality, reliability, and trustworthiness vary significantly across different sources. Our work addresses this challenge by integrating adaptive AI-based evaluation into industrial sensor applications, ensuring that measurement data is dynamically weighted and refined for optimal decision-making.
The Challenge: Information Overload and Trustworthiness
Digitalisation has made vast amounts of sensor data available across various industries. However, not all data is of equal value. Data quality varies based on sensor accuracy, environmental conditions, and even subjective human input and handling. In addition, the rise of AI-generated data and adversarial AI (e.g., fake or manipulated data) makes it increasingly difficult to determine which data points are truly reliable.
Traditional AI models typically treat all data equally, potentially resulting in sub-optimal predictions and derived decisions. Current approaches, such as physics-informed models, incorporate fundamental scientific principles (e.g., differential equations) to improve AI-based evaluations. Our work extends this concept by introducing curator-informed models that systematically integrate expert knowledge, data provenance, and real-time context into AI-driven measurement systems.
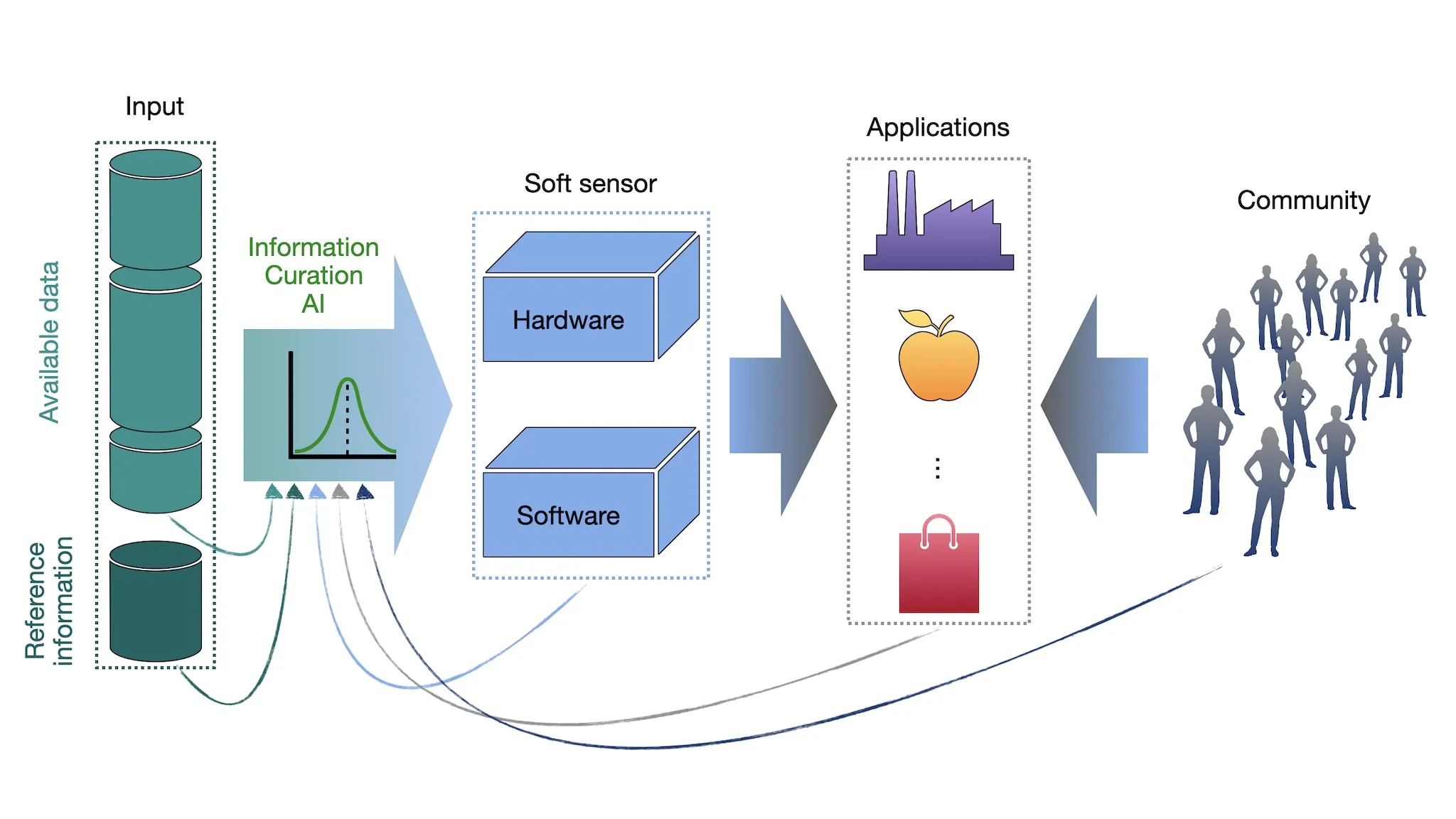
Scientific and Technological Innovation
Compolytics’ curator-informed models go beyond conventional sensor fusion techniques by dynamically evaluating data quality based on:
- Reliability & Trustworthiness – Assigning confidence scores to sensor data based on historical accuracy and contextual relevance.
- Dynamic Valuation – Adjusting the importance of data over time, ensuring that outdated or low-quality data does not compromise results.
- Relearning & Forgetting – Enabling AI systems to continuously update and refine models based on evolving measurement conditions.
- Traceability & Compliance – Aligning with Article 43 of the EU Artificial Intelligence Act and further legal requirements, ensuring interpretability and regulatory compliance.
Our approach uniquely incorporates negative data, meaning that AI models learn not only from correct data but also from errors, uncertainties, and inconsistencies. This multi-source integration allows to process high-precision sensor data alongside heterogeneous, lower-fidelity datasets in our workflows – a breakthrough in industrial AI and measurement technology.
Industrial Applications and Market Potential
The integration of curator-informed models into sensor-based industries unlocks new levels of precision and efficiency, making it a transformative technology for agriculture and food processing, lifestyle applications, as well as sensor-assisted traceability.
The market for data-driven solutions in these industries is expected to grow substantially in the coming years. This will be driven by new application fields, changing consumer expectations, and regulatory requirements for transparency and traceability of technical solutions, as well as AI reliability and security.
Shaping the Future of AI in Measurement Technology
Our research establishes a new standard in AI-assisted sensor technology by systematically improving data evaluation and integration. With the introduction of curator-informed models, Compolytics is setting new guidelines for trustworthy, application-specific AI systems that enhance industrial, environmental, and consumer applications.
By bridging AI research with practical industrial applications, we are making sensor data smarter, more adaptive, and more valuable – ensuring that our customers can rely on their measurements with confidence when using Compolytics’ sensing solutions.
This project is partly funded by the European Union.
Funding period: 1st Jan 2025 – 31st Dec 2027.
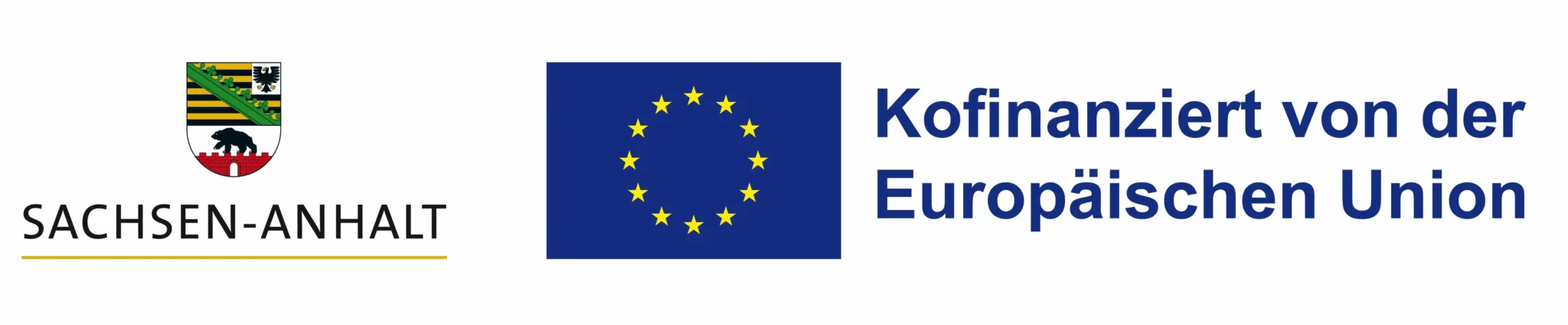